Major research outcome
- Ph.D. Candidate Yedam Lee and Professor Sang Lee develop urban turbulence statistics prediction technique based on recurrent neural networks
- 관리자 |
- 2025-03-27 15:52:51|
- 31
Urban Air Mobility (UAM) is an emerging air transportation system that utilizes eco-friendly electric vertical take-off and landing vehicles (eVTOLs) and vertiports to transport people and cargo safely and efficiently within urban areas. Due to their smaller size compared to conventional aircraft, UAM vehicles are highly sensitive to localized meteorological phenomena occurring in urban environments. Therefore, the study and prediction of urban airflow are essential to ensure the safe operation of UAM systems. Moreover, to minimize the impact of rapidly changing urban flows and microclimatic effects on UAM operations, high-accuracy predictions with near real-time performance are required. However, conventional CFD-based urban flow simulations are limited in their applicability for real-time predictions due to their long computation times and high computational costs.
Ph.D. candidate Yedam Lee and Professor Sang Lee have developed a high-speed urban boundary layer flow prediction model by integrating artificial intelligence techniques based on recurrent neural networks with reduced order modeling (ROM). In this study, a ROM framework preserving over 99.9% of flow energy was constructed to maximize turbulence prediction accuracy. Notably, the Long Short-Term Memory (LSTM) network outperformed the Gated Recurrent Unit (GRU) in prediction performance. The proposed method achieved over 40 times faster computation speed compared to traditional numerical prediction methods, demonstrating its potential applicability in urban aerodynamics and real-time turbulence forecasting.
This study not only highlights the high practicality of the proposed predictive model for real-world urban environments, but also suggests its potential to significantly advance prediction capabilities in urban planning and environmental monitoring. The study was published in the international journal Building and Environment, a globally recognized and authoritative journal in the fields of building science and urban engineering. Building and Environment has a recent Impact Factor of 7.1 and ranks within the top 5% of journals in its JCR category.
Ph.D. candidate Yedam Lee and Professor Sang Lee have developed a high-speed urban boundary layer flow prediction model by integrating artificial intelligence techniques based on recurrent neural networks with reduced order modeling (ROM). In this study, a ROM framework preserving over 99.9% of flow energy was constructed to maximize turbulence prediction accuracy. Notably, the Long Short-Term Memory (LSTM) network outperformed the Gated Recurrent Unit (GRU) in prediction performance. The proposed method achieved over 40 times faster computation speed compared to traditional numerical prediction methods, demonstrating its potential applicability in urban aerodynamics and real-time turbulence forecasting.
This study not only highlights the high practicality of the proposed predictive model for real-world urban environments, but also suggests its potential to significantly advance prediction capabilities in urban planning and environmental monitoring. The study was published in the international journal Building and Environment, a globally recognized and authoritative journal in the fields of building science and urban engineering. Building and Environment has a recent Impact Factor of 7.1 and ranks within the top 5% of journals in its JCR category.
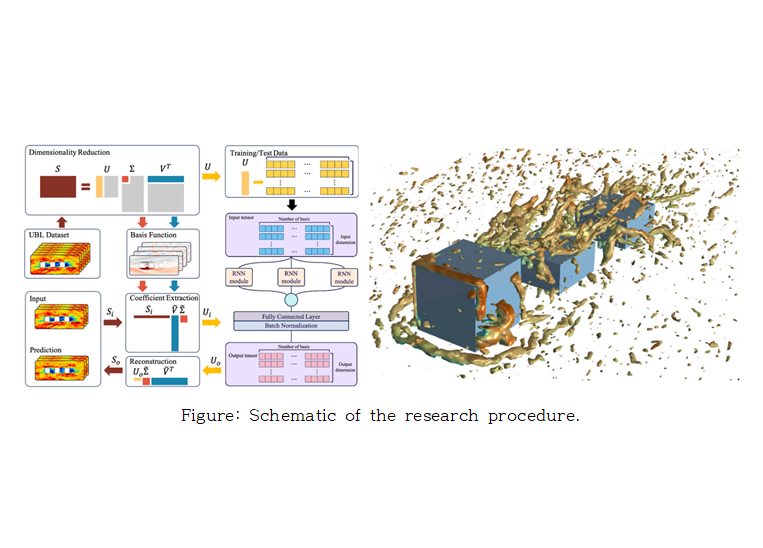
Attach File |
---|